Tanus Bot, Actuarial Analyst – Team Leader, Oak Tree
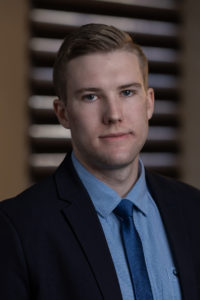
As an analyst who helps clients prepare their data for reinsurance support, I have seen my fair share of inaccurate and un-useable data. The implications of this in the insurance industry run from the man on the street who needs risk protection to the reinsurers covering the mostly small chance of a catastrophe occurring on this person’s policy. From the perspective of a reinsurance broker, the data ultimately has an impact on the price of your reinsurance treaty.
Whilst I don’t believe data is being willfully corrupted for financial gain, I think there can sometimes be some negligence from the data capturers as they may not understand the use case. One example of this being location-based data. Insurers generally don’t hold their catastrophe risk, which is why location-based statistics is not a huge concern to insurers but is essential to reinsurers. Having accurate location based statistics can allow an insurer to manage any accumulation on their risks whether that be through risk management or policy wording.
Another example being, exposure statistics, particularly on large multi-national chains and un-named locations. They will be sold on their largest exposure site and the rest of the exposure is taken to be null. This can obviously lead to accumulations.
Why should insurers care about accumulation? When insurance products are priced, they are priced on the basis of the risks being independent. This allows us to use branches of statistics which we would not have been able to use otherwise. Risks sharing locations or being in the same area may be subject to the same perils and so would not be independent which would invalidate some of the assumptions analytics people have to make.
One of the problems the insurance industry is facing at the moment is the sheer diversity of data among the different providers. If you’ve ever coded a program you would understand the phrase, “garbage in, garbage out”. This generally refers to the validity of the conclusions we are able to draw based on the quality of the data being used. Coding allows users to standardise procedures around analysis so that you can reduce work load and apply the same program to different scenarios. As you can imagine, this can be difficult if all the data you are using in the different scenarios (different clients) is reported differently, has a different layout and has different reporting requirements. It ends up being easier to treat each client on a case by case basis.
Let’s say for instance the accuracy of data is about 70% on a retail level over a whole portfolio (between clients filling out forms, brokers recording information and insurers keeping track of it all). Now say we (the reinsurance broker) are only able to collect/ accurately represent 70% of our client’s data. This would mean only 51% of the data is complete and accurate. You can imagine the impact of this on an insurers treaty and so the importance of cleaning /verifying data. We like to work together with our clients to fight back against bad data and help accurately represent their business in the reinsurance market space! A humble reinsurance brokerage doing their part in the huge value chain that is the insurance industry.