Nick Feast from SAS.
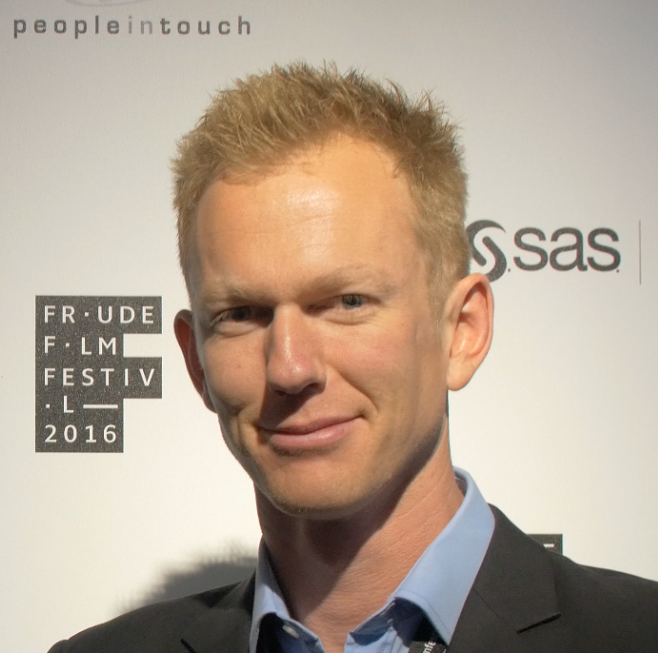
Combating fraud in the insurance industry is a complicated process, facing significant challenges. I would like to share some insights into how analytics solutions are helping the industry in this task.
Some background – SAS has been a pioneer in analytics for over 45 years, starting with agricultural data in the seventies. Today, we offer around 400 products that address various business challenges across numerous industries, including pharmaceuticals, construction, marketing, retail, and financial services. Fraud and financial crime detection and regulatory compliance have become critical growth areas for us, particularly in the banking and insurance sectors.
A Dual Approach to Fraud Detection and Prevention – Fraud affects all industries, and the insurance sector is no exception. Detecting and preventing fraud is essential to protect both the insurer and the customer. In insurance, this means scrutinising claims for suspicious activity and assessing the risk of new applications at the onboarding stage.
Insurers face a tough balancing act: they need robust controls to identify fraudulent claims whilst also ensuring genuine claims are processed swiftly and efficiently. Traditional processes are manual, resource-intensive and often ineffective, as no organisation can review every claim in detail. This is where analytics comes in, automating the detection process and allowing experienced assessors to focus on the most suspicious cases.
The Role of Analytics – Analytics enhances detection capabilities by identifying patterns and trends that manual processes might miss. It involves using a combination of techniques, including predictive models, anomaly detection, business rules, text analytics, and advanced network analytics. This layered approach improves detection accuracy and reduces false positives, ensuring that investigators only work on high-quality alerts.
Enhancing detection and prevention with AI and Machine Learning:
AI has revolutionised fraud detection and prevention by enabling faster, more accurate decision-making.
Supervised learning involves training models on labeled data to predict the likelihood of fraud. For example, we might use a year’s worth of claims, which are labeled as either fraudulent or legitimate, in order to train a model. This model can then be used to identify new claims that are likely to be fraudulent, based on historical patterns. These models are continuously updated to adapt to new fraud tactics.
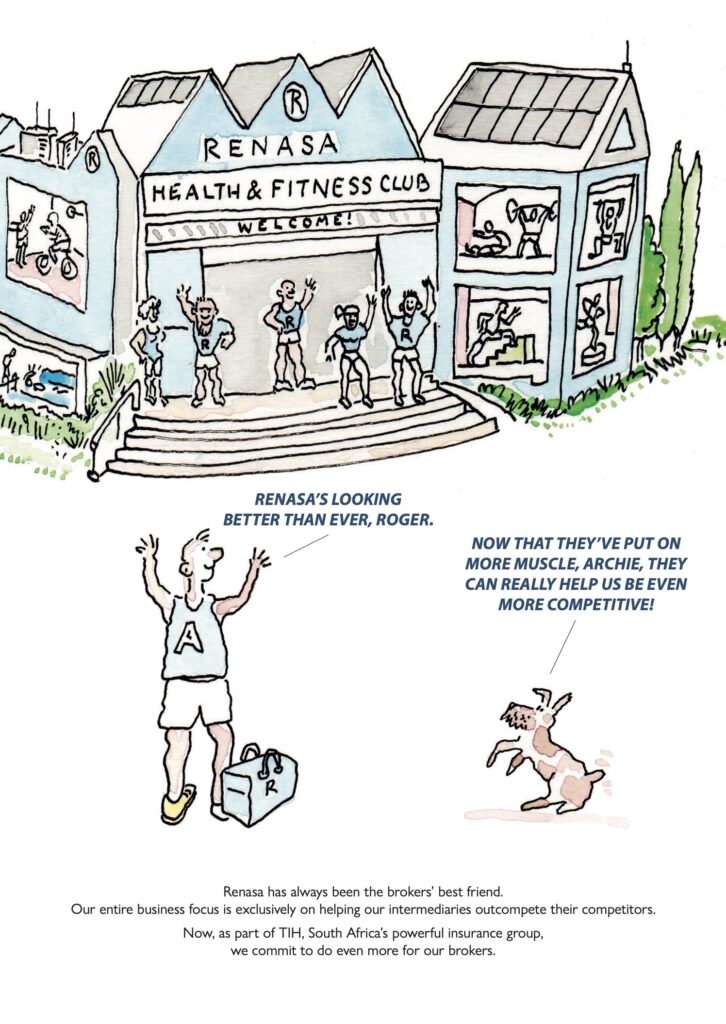
Renasa has always been the broker’s best friend.
Our entire business focus is exclusively on helping our intermediaries outcompete their competitors.
Now, as part of TIH, South Africa’s powerful insurance group,
we commit to do even more for our brokers.
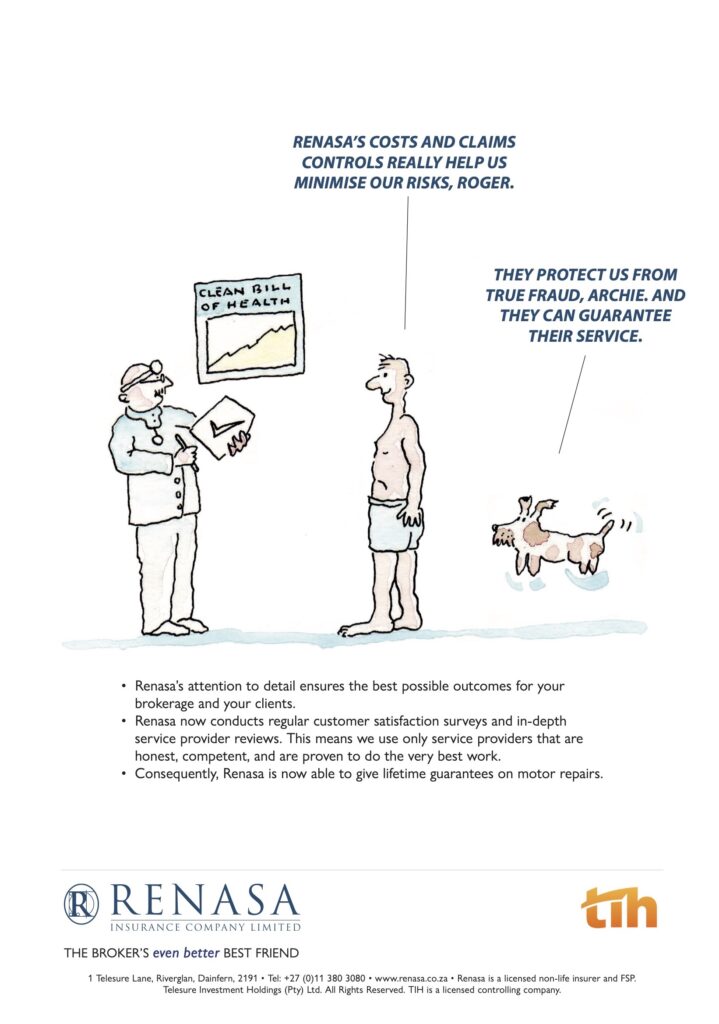
Renasa has always been the broker’s best friend.
Renasa’s attention to detail ensures the best possible outcomes for your brokerage and your clients.
- Renasa now conducts regular customer satisfaction surveys and in-depth service provider reviews. This means we use only service providers that are honest, competent, and are proven to do the very best work.
- Consequently, Renasa is now able to give lifetime guarantees on motor repairs.
Renasa is a licensed non-life insurer and FSP. Telesure Investment Holdings (Pty) Ltd. All Rights Reserved. TIH is a licensed controlling company.
In contrast, unsupervised learning, or anomaly detection, builds a profile of normal behavior and identifies deviations from this norm. This is particularly useful in scenarios where there is a lack of labeled data available for building models but can also be very effective at identifying new types of fraudulent activity. By detecting anomalies, insurers can uncover suspicious behavior that might otherwise go unnoticed.
Hybrid Approach
A hybrid approach, combining predictive models, anomaly detection, business rules, and network analytics, offers a comprehensive solution. For instance, social network analysis can identify relationships and nodes of influence, revealing hidden connections and collusion between disparate entities, as well as other patterns and associations that may be indicative of fraud. This method considers both individual entities and the broader network, providing a powerful tool for both fraud detection and investigation.
Data Quality: The Foundation of Effective AI – Effective fraud detection and prevention rely on high-quality data. At SAS, we assist organisations in preparing their historical data for AI modeling and fraud detection. This involves a range of data quality and standardisation processes, including entity resolution, which helps to consolidate information across multiple sources and build accurate entities and networks. Without good data, even the best analytics solutions will fail so this step is a crucial part of the process.
Beyond Fraud Detection – Our solutions extend beyond fraud detection to other areas of the insurance industry, including underwriting, pricing, and actuarial modeling. Many of the AI and analytical techniques used for fraud detection can be applied to predict customer behavior, optimise pricing strategies, and improve risk assessment.
The insurance industry is on the cusp of a technological revolution. AI and advanced analytics are transforming how insurers detect and prevent fraud, ultimately leading to more efficient and secure processes. At SAS, we are committed to helping our clients navigate this brave new world, providing the tools and expertise needed to stay ahead of emerging threats.