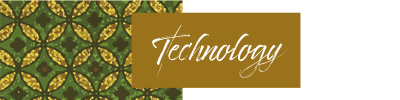
Amber Sutherland, Silent Eight
A banking insider’s guide to the 5 questions you should ask (and why).
After almost a decade working in a large, global bank, I can speak to the challenges faced by all three lines of defense in trying to combat financial crime. I can also attest to the effect these processes had on our clients. As a front-line corporate relationship manager, I frequently had to navigate the KYC, remediation and payment screening process for my clients.
Not only was this an incredibly time consuming and frustrating process on an organizational level, but more painful was the deleterious effect it had on our clients and their business: crucial payments to vendors were delayed unnecessarily, accounts took months to open, and required incessant back and forth between multiple parties, and account fundings/transactions always came down to the wire because of basic due diligence, regardless how much work you tried to do ahead of time.
Much of the process that required our intervention seemed mundane, repetitive and inefficient, which compounded everyone’s frustration.
Sound familiar?
These types of repetitive, mundane tasks are ideally suited to be outsourced to artificial intelligence, which the industry seems to now realize.
Artificial intelligence can be an incredibly valuable tool, in that it can offload mundane tasks, provide insight into customer and employee behaviour, create more standardization, and help reduce or manage costs.
But as technology becomes increasingly sophisticated, there are many factors to weigh in the decision making process.
After countless conversations with stakeholders and decision makers in the industry, I have learned that there are 5 main concerns when implementing regulatory technology, especially AI technology, in the financial sector.
I’ve outlined them below, along with why they matter:
- How transparent is the AI?
- What if the AI learns the wrong behaviours, such as bias?
- Does it have more than one purpose? What is the roadmap?
- Is it better than what I have now? More accurate, faster, more standardized, more cost effective? Can ‘better’ be tested quantifiably?
- What are the redundancies? How will this technology affect my operational resiliency?
Let’s look at each point in order.
- How transparent is the AI?
While this seems like a straightforward question, “transparent” really encompasses three separate factors:
- Will my team and our stakeholders be able to understand how it works?
- Will I be able to easily demonstrate to Audit, the Board and regulators that it’s doing what it’s supposed to do?
- Can I get a snapshot of what is happening at any given moment?
All of the major regulators have stipulated that artificial intelligence solutions be explainable and demonstrable. Both of these concepts are rather self-explanatory but still worth exploring.
Explainability
It’s not sufficient for your compliance team to understand how the AI makes decisions. They also need to be comfortable explaining the process to key stakeholders, whether they are board members, the internal model committee, audit, or the regulators.
If your technical team can’t understand the technology or how decisions are made, or if the vendor claims confidentiality to protect their IP, this is a cause for concern.
Demonstrability
Like transparency, demonstrability captures a few components – it means you have to be able to demonstrate:
- What decisions the AI has made;
- What changes you’ve made to how the AI makes decisions; and
- Who made the changes.
This is where an audit trail comes into play. First of all, is there one? And if so, is it immutable, and does it capture all actions in the AI or just some of them? Is it exportable in report format and, if so, is the report readable and can it be easily understood?
Compliance is a data-driven world, and the risk associated with being deemed non-compliant is substantial. Being able to capture and export changes to, and decisions made within your AI is crucial to your relationships with your stakeholders.
As personal liability expands in the corporate world, board members and committees increasingly require an understanding of not only how compliance risk is being mitigated, but also clear evidence that it’s being done, how and by whom.
2. What if the AI learns the wrong behaviours, such as bias?
The underlying questions here, without detracting from the very serious concern about embedding existing unconscious bias into your AI, are as follows:
- If the AI is wrong, or my requirements change, can I fix it? How easily?
- What impact will tweaking the AI have on everything it’s already learned?
An industry journalist recently asked me if I thought bias was a problem with AI. My answer to her, and to all of you, is that AI simply learns what’s already happening within your organization. As a result, unconscious bias is one of the things that AI can learn, but it doesn’t have to be a problem.
While you can’t really prevent AI from learning from past decisions (that’s kind of the point), good technology should enable you to identify when it’s learned something wrong, and to tweak it easily to prevent bad decision-making from becoming embedded into your AI’s decision making.
This ties in to the need for transparency and reporting. It’s not only necessary to see how decisions are made, you also need to be able to prevent poor decisions or bias from being part of the AI’s education. And all of these things need to be documented.
When testing out new vendors, once the AI engine has been trained initially for your proof of concept, you should be able to clearly understand the findings, and be able to make changes at that time (and thereafter). You will very likely be surprised by some of the ways decisions are currently being made within your organization.
For example, at Silent Eight, our technology investigates and solves name and transaction alerts for banks. This work is typically done by teams of analysts, who investigate these alerts, and close them as either a true positive (there is risk here) or false positive (there is no risk). True positive alerts require substantially more time to investigate and close than alerts deemed to be false positive.
Analysts typically have KPIs around the number of alerts they’re expected to investigate and close each week.
By late Friday, the analysts are doing everything they can to make sure they meet this quota. As a result, it’s not unusual during the AI training process that the AI learns that 4pm on Fridays is a great reason to close out pending alerts as false positives.
Obviously this is a good example of AI learning the wrong behaviour and needing to be tweaked. It’s also a good example of mistaking correlation with causation, which is a topic worthy of its own examination on another day.
Today, as regulations are introduced and amended, you’re continually updating your policies to reflect these changes. It’s no different with artificial intelligence. It’s imperative that your AI engine is correspondingly easy to tweak, and that when you tweak it, you don’t lose everything it has already learned.
Thoughtful, well-architected technology should be built in a manner that makes it easy to update or amend part of the AI engine, without impacting the rest of the learnings. This is something you should both ask about, and test in the POV environment.
3. Does the AI have more than one purpose? What is the roadmap?
Many financial institutions have the dueling mandates to be both innovative and transform digitally, but also rationalize vendors. So, when considering artificial intelligence solutions, which are often niche, it’s worthwhile finding out:
- How the vendors decide to build out features;
- Whether they are willing to customize their offering for you;
- How reliably they’ve delivered on features in the past; and
- Whether what’s on their roadmap adds value for you.
This way you can ensure that the decision you’re making is one that is future-proofed and set up for longevity.
4. Is it better than what you have now?
Better can mean different things to different organizations and individuals. It’s typically tied into the problems you’re experiencing now, and what your organization’s strategic focus and priorities are. When I ask clients and prospects what they mean by ‘better’ the answers I hear most commonly are:
- Is it more accurate?
- Is it faster?
- Will it give me greater standardization?
- Will it enable me to identify more risk?
- Will it enable me to federate by jurisdiction?
- Will it lead to greater efficiencies?
- Is it more cost-effective?
- Does it increase my visibility? Ie. is it transparent?
Once you’ve defined what ‘better’ means to you and your organization, you need to find out from your prospective vendors if and how ‘better’ can be tested quantifiably.
5. What are the third-party dependencies? How will this technology affect my operational resiliency?
Operational resiliency and third party due diligence have become a significant focus in the industry and can be a barrier to doing business. Many regulators, including the EBA and the FCA, have issued guidelines on the topic, and continue to revisit it
It’s vital to understand if a vendor is reliant on any other vendors in their tech stack, if they’re using open source code, what their deployment is (on-premise, in the cloud, in a private cloud), and what security standards they adhere to.
Take back the things you can control
Right now, the financial services industry is beset by many challenges that are outside of its control, including the low-interest environment, working remotely, bad debt provisions, and the increased new accounts and suspicious activity resulting from COVID.
Your compliance costs and processes are a piece of the puzzle you can control. Good artificial intelligence technology will enable you to offload some of your mundane, repetitive tasks, freeing up you and your team to focus on more complex risks and higher-value projects.
I recognize that artificial intelligence can be a bit daunting and that it has a mixed reputation in the industry. However, if you’re armed with a dose of skepticism, the right questions to ask, and you approach it with an open mind, you’ll be amazed by what it can do.
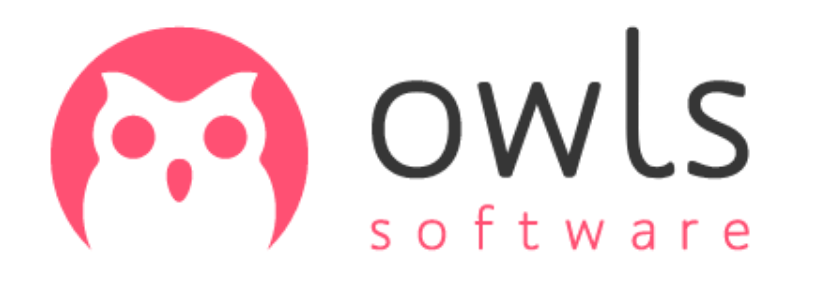
At OWLS Software we offer 8000 insurance software features designed to automate any Insurers, UMAs or Brokers insurance admin.